How to Implement LLM Observability for Production (Part 2)
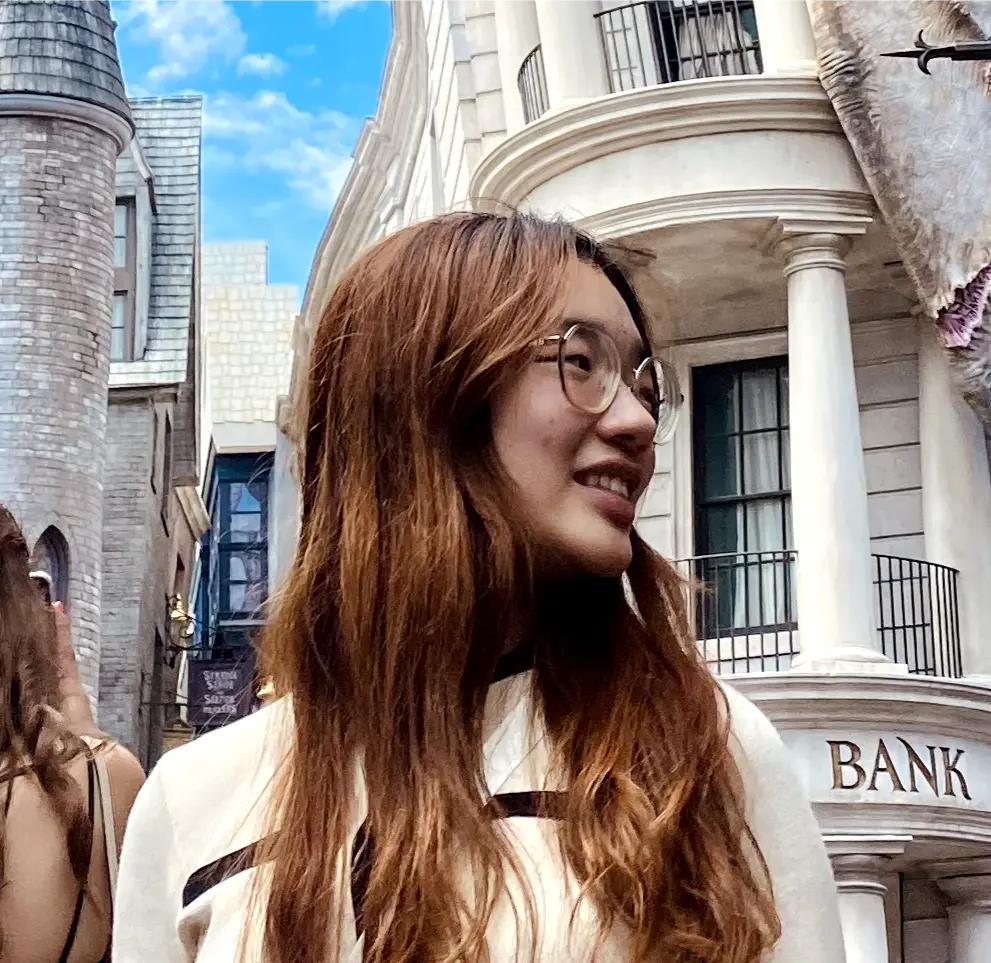
In our previous article, we explored the fundamentals of LLM observability and why it's crucial for building reliable AI applications. Now, let's dive into the practical side-how to actually implement effective monitoring for your LLM applications.
Best Practices for Monitoring LLM Performance
1. Using Prompting Techniques to Reduce Hallucinations
LLMs sometimes generate inaccurate outputs that sound plausible - also known as hallucination. Hallucinations can happen frequently and undermine your user's trust.
The good news is, you can mitigate this by using the right prompting technique.
For example:
- Use chain-of-thought to prompt the model to adopt step-by-step reasoning.
- Ground the model responses in trusted external knowledge using RAG.
- Structure the output format to limit the model's freedom to hallucinate.
- Give few-shot examples for the model to follow.
We wrote about other prompting techniques, too. Another useful feature in Helicone is being able to experiment with your prompts with production data.
2. Preventing Prompt Injections
Malicious users can manipulate their inputs to trick your model into revealing sensitive information or take risky actions. But there are ways to prevent this.
On a high-level, you can:
- Sanitize inputs by removing special characters and injection patterns.
- Implement strict validation of user inputs.
- Block inappropriate or malicious responses.
- Monitor inputs using observability tools like Helicone and flag suspicious activities.
We dive deeper into this topic in this blog: How to prevent prompt injections.
3. Caching to Improve Performance and Latency
Caching stores previously generated responses, allowing applications to quickly retrieve data without additional computation.
In most use cases, latency has the most impact on the user experience. Helicone allows you to cache responses on the edge, so that you can serve cached responses immediately without invoking the LLM API, reducing costs at the same time.
4. Tracking API Usage and Costs
It's important to know exactly what is drilling a hole in your operational cost.
In Helicone, you can actively track the cost incurred for every LLM interaction. We help you answer questions like:
- Which model is driving your costs?
- Is the premium justified?
- Which users are generating the highest costs? Are power users subsidizing occasional users?
- Which features have the highest LLM spend? Is the ROI worth it?
There are ways to optimize your LLM costs:
- Monitoring LLM costs by project or user.
- Use cheaper model for simpler tasks, and thinking models where quality is more important than latency.
- Set rate limits for certain users or features
- Caching common responses (see #3 above)
For more effective cost optimization strategies, check out this blog.
5. Iterating on the Prompt
As models evolve, it's important to continuously test and audit your prompts to ensure they're performing as expected.
You should experiment with different variations of your prompt, switch models or set up different configurations to find the best performing prompt. You should also evaluate against key metrics that's important to your business.
Getting Started with LLM Observability
Step 1: Choose Your Integration Method
Helicone offers two primary integration methods:
-
Proxy Integration: The simplest approach that requires minimal code changes:
import OpenAI from "openai"; const openai = new OpenAI({ apiKey: process.env.OPENAI_API_KEY, baseURL: `https://oai.helicone.ai/v1/${HELICONE_API_KEY}/` });
-
Async Integration: Direct logging without modifying your API endpoints.
Helicone supports any provider and framework. For other integration methods, check out the docs.
Step 2: Add User and Session Tracking
Enhance your data with user and session tracking for better segmentation. This allows you to get deeper analytics per user, or per conversation for debugging purposes.
const sessionId = generateSessionId();
const response = await openai.chat.completions.create({
model: "gpt-4",
messages: chatHistory,
headers: {
"Helicone-User-Id": userId,
"Helicone-Session-Id": sessionId
}
});
Step 3: Implement Custom Properties
Add other specific metadata (aka. custom properties) to your requests for further data segmentation. For example, you can track feature flags, user roles, or source of the request.
const response = await openai.chat.completions.create({
model: "gpt-4",
messages: chatHistory,
headers: {
"Helicone-Property-Feature": "product_recommendation",
"Helicone-Property-User-Segment": "premium",
"Helicone-Property-Source": "mobile_app"
}
});
That's the basic setup! You can learn more about the different integration methods and features in Helicone in the docs.
Effective LLM Observability Tools
As companies rush to integrate LLMs into their business functions, observability platforms have transitioned from basic logging to comprehensive platforms that support the entire LLM lifecycle.
Helicone is an open-source LangSmith alternative with impressive 2.3 billion processed requests, 3.2 trillion logged tokens, and 18.3 million tracked users. Other popular tools include Portkey, Langfuse, and Traceloop.
Comparison of Popular Observability Tools
Feature | Helicone | LangSmith | Langfuse | Portkey |
---|---|---|---|---|
Ease of Integration | Simple proxy or async | SDK integration | SDK integration | Proxy or SDK integration |
Open Source | ✅ | ❌ | ✅ | ✅ |
Self-Hosting | ✅ | Enterprise only | ✅ | ✅ |
Multi-Provider Support | All provider and framework | Optimized for LangChain | All provider and framework | All provider and framework |
Caching | ✅ | ❌ | ❌ | ✅ |
Cost Tracking | Detailed | Limited | Limited at scale | Detailed |
Security Features | Advanced | Basic | Basic | Requires extra setup |
Data Segmentation | Advanced | Limited | Limited | Limited |
Enterprise Support & Compliance | ✅ | ✅ | ✅ | ✅ |
These monitoring tools provide the visibility developers need to monitor, debug, and continuously improve their AI applications.
Time to Take Action!
Now that you have a good understanding of how to implement monitoring strategies, it's time to put them into practice! We recommend signing up with a platform mentioned above, start logging, and see how users are interacting with your LLM app.
We are here to help you every step of the way! If you have any questions, please reach out to us via email at support@helicone.ai or through the chat feature in our platform. Happy monitoring!
You might find these useful:
- Why You Should Monitor Your AI Applications (Part 1)
- How to Reduce LLM Hallucinations
- How to Test Your LLM Prompts (with Helicone)
Questions or feedback?
Are the information out of date? Please raise an issue or contact us, we'd love to hear from you!